Electricity theft is a significant challenge for power utilities worldwide, leading to substantial financial losses and compromising grid reliability. Traditional detection methods often fall short in identifying sophisticated theft techniques. However, advancements in Artificial Intelligence (AI) have introduced innovative solutions to effectively detect and mitigate electricity theft.
Understanding Electricity Theft
Electricity theft involves unauthorised consumption of electrical power through methods such as illegal tapping of power lines, bypassing or tampering with meters, and manipulating consumption data. These activities not only result in revenue losses but also pose safety hazards and disrupt equitable energy distribution.
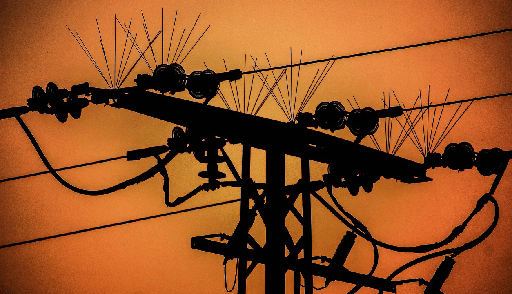
AI-Powered Detection Methods
AI enhances electricity theft detection through several approaches:
- Machine Learning Algorithms: AI models analyse consumption patterns to identify anomalies indicative of theft. For instance, a study proposed an adaptive time-series recurrent neural network (TSRNN) combined with the Synthetic Minority Over-sampling Technique (SMOTE) to detect abnormal usage patterns, achieving a discriminant accuracy of 95.1%.
- Deep Learning Techniques: Advanced models like Long Short-Term Memory (LSTM) networks predict energy consumption and compare it with actual usage to detect discrepancies. The GrAb model utilises LSTM for consumption prediction and a Support Vector Machine (SVM) classifier to categorise energy losses, effectively identifying various forms of theft.
- Ensemble Methods: Combining multiple AI models enhances detection accuracy. The EnsembleNTLDetect framework employs stacked auto-encoders for dimensionality reduction and a soft voting ensemble classifier to detect aberrant consumption patterns, demonstrating improved performance over traditional methods.
- Privacy-Preserving Models: Addressing data privacy concerns, federated learning systems train detection models without exchanging raw data. This approach maintains user privacy while effectively identifying theft.
Challenges and Future Directions
Despite advancements, challenges persist, including:
- Data Imbalance: The scarcity of labelled theft data complicates model training. Techniques like data augmentation and synthetic data generation are being explored to address this issue.
- Evolving Theft Techniques:As theft methods become more sophisticated, AI models must continuously adapt. Incorporating real-time data and adaptive learning mechanisms is crucial.
- Integration with Existing Systems: Seamlessly integrating AI solutions into current infrastructure requires careful planning to ensure compatibility and effectiveness.
AI-Powered Precision
Ongoing research focuses on developing more robust AI models capable of handling diverse and complex theft scenarios, ensuring the security and efficiency of power distribution systems.